Last Updated on 30 Dec 2024
How Does Device Fingerprinting Work?
Share in
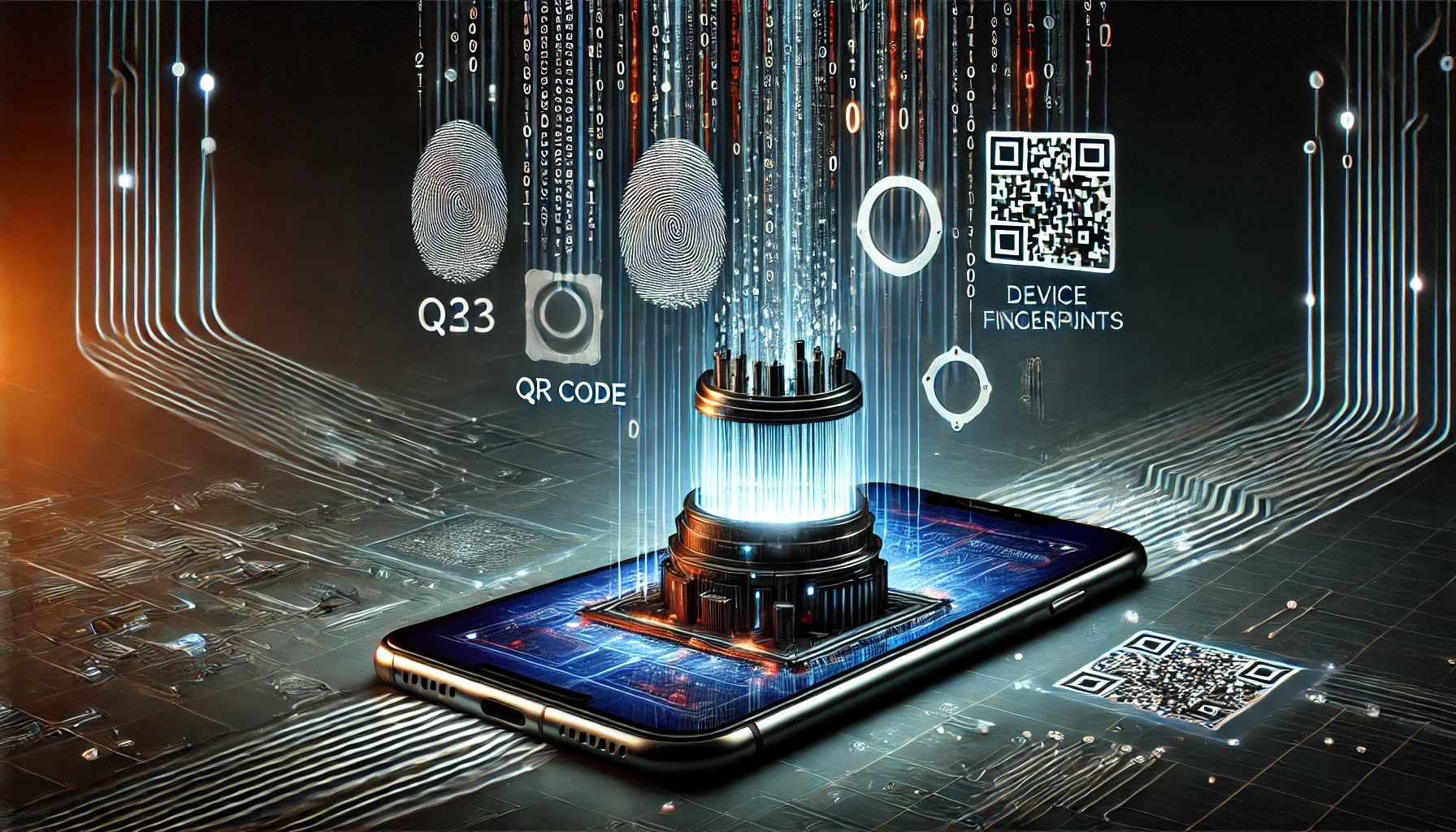
Key Notes:
•
Privacy-Centric Design:Device fingerprinting balances precision and privacy through selective data collection, anonymization, and GDPR compliance, ensuring security and trust.
•
Comprehensive Data Collection:Device fingerprinting gathers hardware, software, and behavioral data—such as screen resolution, OS details, and interaction patterns—to create precise, persistent identifiers.
•
AI-Powered Future:AI and ML enhance fingerprinting with continuous learning and real-time fraud detection, adapting to evolving threats.
Introduction
In today's digital landscape, where fraud tactics are becoming increasingly sophisticated, businesses must adopt equally advanced tools to safeguard their platforms. Device fingerprinting, explored in depth through its various aspects in this blog post, has emerged as a groundbreaking technology in this domain, offering precise, persistent identification of devices by analyzing a wide range of attributes. But how does it achieve such accuracy, and why is it so effective in fraud detection?
This blog unpacks the mechanics of device fingerprinting, highlighting the advanced techniques that make it a cornerstone of modern cybersecurity. From behavioral analysis to AI-driven adaptability, you'll discover how this technology works, what data it collects, and its transformative role in combating fraud while respecting user privacy.
How Device Fingerprinting Works?
Device fingerprinting creates a unique digital identifier for a device by analyzing a combination of its attributes. These attributes are collected from the device’s hardware, software, and network environment, enabling precise and persistent identification. Here’s a simplified breakdown of the process:
•
Collecting Features: Basic attributes such as the device model, operating system, screen resolution, and network settings are gathered. Additional data, like browser type or installed plugins, further refine the fingerprint.•
Creating a Unique Identifier: Advanced algorithms combine these attributes to generate a "fingerprint" that uniquely identifies the device across sessions.•
Adapting to Changes: When device configurations change, resilient techniques, such as similarity-based hashing, analyze relationships between old and new data to maintain accurate identification.•
Enhancing with Context: Incorporating the relationship between devices and accounts adds another layer of accuracy, ensuring robust recognition in dynamic environments.
The method is comparable to recognizing a person by their unique handwriting or habits—it adapts to changes while maintaining accuracy over time. This is particularly useful for preventing fraud, enhancing security, and ensuring reliable user authentication.
What Information Is Collected to Create a Device Fingerprint?
Considering the user's privacy concerns, device fingerprinting relies on a combination of hardware, software, and network attributes to create a unique identifier for each device. Here’s a concise list of the most commonly used data points:
•
Browser Information
•
Browser Type and Version:E.g., Chrome 116, Safari 17.
•
User Agent String:A text that describes the browser, operating system, and device.
•
Installed Plugins and Extensions•
Cookies and Local Storage:Tracks browser-stored data for session management.
•
Operating System Details
•
OS Type and Version:E.g., Windows 10, macOS Ventura, Android 13.
•
Language and Region Settings:Reflects geographic and cultural preferences.
•
Time Zone:Indicates the device’s physical or virtual location.
•
Hardware Specifications
•
Screen Resolution:The dimensions of the device display (e.g., 1920x1080).
•
Device Model and Manufacturer:E.g., iPhone 14 Pro, Dell XPS.
•
CPU and GPU Details:Includes processor and graphics card information.
•
Touchscreen Capabilities:Determines if the device has a touchscreen.
•
Network Attributes
•
IP Address:Helps approximate the device’s location.
•
Wi-Fi or Cellular Network Details:Provides connectivity context.
•
Network Speed and Latency:May indicate the device's usage patterns or location
•
Interaction Patterns (Behavioral Data)
•
Mouse Movements and Clicks:Tracks user interaction on non-touch devices.
•
Touchscreen Gestures:Captures swipes and taps for touch-based devices.
•
Keyboard Typing Speed:Monitors patterns for additional identification.
•
Advanced Fingerprinting Techniques
•
Canvas Fingerprinting:Uses the rendering of 2D graphics to generate a unique signature.
•
Audio and Video Capabilities:Checks for microphones, speakers, or cameras.
•
WebRTC Data:Reveals device connectivity and network interfaces.
Real-World Testing Scenarios for Fingerprinting
Developing robust and adaptive device fingerprinting systems presents significant challenges, primarily due to the absence of standardized benchmarks in this domain. This lack creates two key issues:
•
Lack of training datasets:Without standardized datasets, supervised algorithms in fingerprinting lack the necessary data to train effectively, reducing their accuracy and adaptability.
•
Lack of evaluation datasets:The absence of consistent datasets for testing makes it difficult to compare different fingerprinting methods, preventing fair evaluation and benchmarking.
To address these challenges, device fingerprinting tools like CrossClassify employ carefully designed testing scenarios that simulate real-world conditions. These scenarios replicate dynamic environments, such as device updates, network changes, and multi-device usage, providing a controlled yet diverse framework for testing. This approach allows tools to adapt, detect anomalies, and maintain accuracy despite variability, ensuring reliable and effective fingerprinting. In this section, we outline key test scenarios, including examples from CrossClassify's comprehensive test package, demonstrating how these solutions bridge the gaps caused by the lack of standardized datasets.
•
Browser Changes
Devices frequently update or switch browsers, altering attributes like version numbers, plugins, or user agents. Robust fingerprinting systems must recognize the same device even after such changes (you can see one browser test scenario from here)Example: A user accesses a banking site in incognito mode. The fingerprinting system still identifies the device by analyzing attributes like screen resolution, hardware specifications, and operating system details, ensuring account security.
•
Location Modifications
Devices often connect from different networks, whether at home, work, or public spaces. This can alter network-related attributes like IP addresses and geolocation data (See the CrossClassify location modifications test scenario here)Example: A user logs into their account from a café in another city. The system recognizes the device by combining hardware and software attributes, flagging the new location for verification without blocking access unnecessarily.
•
Multi-Device Usage
In shared environments, multiple devices might use the same account, or a single device might access multiple accounts. Fingerprinting systems must distinguish between legitimate and suspicious activities (See the CrossClassify multi-device usage test scenario here)Example: Two family members log into the same streaming account using a tablet and a smartphone. The system identifies both as legitimate devices but flags a third login attempt from a previously unseen device for additional verification.
•
Network Variations and VPN Usage
Users frequently change networks or use VPNs, which can mask or alter network-related attributes. Effective systems maintain accuracy by focusing on hardware and software characteristics instead of relying solely on network data (See the CrossClassify VPN usage test scenario here)Example: A user enables a VPN to access content securely. The fingerprinting system still recognizes the device through persistent attributes like browser settings and device configuration.
Privacy-Preserving Fingerprinting Approaches
Modern device fingerprinting solutions are evolving to prioritize user privacy while maintaining robust and reliable identification capabilities. By adopting selective data collection practices and adhering to strict privacy standards, these solutions can ensure user trust and compliance with global regulations like GDPR and CCPA.
•
Selective Data Collection: Less is More
Instead of gathering extensive data, privacy-preserving fingerprinting focuses on collecting fewer, less invasive features. By relying on attributes that are essential for creating a unique identifier—such as hardware specifications, basic browser details, and operating system settings—solutions can achieve accuracy without intruding on user privacy.
Example: A fingerprinting system might skip sensitive data, like precise geolocation or stored cookies, and instead rely on generic details like screen resolution, device model, and time zone.
•
Anonymization and Encryption
To protect user privacy during fingerprinting, anonymizing and encrypting the collected data is critical.
•
Anonymizationensures that the data cannot be traced back to a specific user or device.
•
Encryptionprotects the data during storage and transmission, safeguarding it from unauthorized access or breaches.
Example: A hashed representation of device attributes can be stored instead of raw data, making it impossible for anyone to reconstruct sensitive details.
•
Gaining User Consent
Transparency is essential when collecting data for device fingerprinting. Users should be informed about what data is being collected, why it is needed, and how it will be used. Obtaining explicit consent ensures compliance with privacy regulations.
What if the user says no? If a user declines to provide consent for fingerprinting:•
Fallback Mechanism:The system can revert to less intrusive methods, such as session-based cookies, which are limited in scope and temporary.
•
Limited Service Access:In cases where fingerprinting is essential for security (e.g., online banking), users may be offered limited access or alternative security options, such as one-time passwords or biometric authentication.
Example: A user accessing an e-commerce platform without enabling fingerprinting might experience reduced personalization but still have access to basic shopping features.
•
Compliance with Privacy Regulations
Fingerprinting solutions must align with regulations like GDPR and CCPA, which emphasize:
•
Data Minimization:Collect only what is necessary.
•
User Rights:Allow users to opt out or request deletion of their data.
•
Purpose Limitation:Use the data strictly for its intended purpose, such as fraud prevention or security.
How Advanced Device Fingerprints Revolutionize Fraud Detection
As fraud tactics evolve, so must the tools used to counteract them. Advanced and highly accurate device fingerprinting plays a pivotal role in enhancing fraud detection by offering deeper insights and unparalleled precision. By leveraging a broader range of device attributes and behavioral patterns, these sophisticated fingerprints go beyond basic identifiers to create a detailed profile of each device, improving the ability to detect fraudulent activities with greater reliability.
•
Enhanced Anomaly Detection
Advanced device fingerprints capture a wide array of attributes—such as hardware configurations, software versions, browsing behaviors, and even usage patterns. This comprehensive dataset enables systems to identify subtle anomalies that indicate fraud, such as a device suddenly operating in a way that contradicts its historical behavior. For example:
•
A legitimate user’s device may undergo routine changes, like a software update, which advanced fingerprinting can account for.
•
Conversely, a fraudster attempting to mimic a trusted device often fails to replicate all attributes precisely, triggering red flags.
•
Detecting Sophisticated Fraud Schemes
Accurate fingerprints can uncover hidden connections between devices and accounts. For instance:
•
Multi-account abuse: Fraudsters using a single device to create numerous fake accounts can be flagged by recognizing the shared fingerprint.
•
Account takeovers: A stolen credential may be used from a device that differs from the original user’s fingerprint, allowing systems to block unauthorized access preemptively.
•
Reducing False Positives
Improved accuracy ensures that legitimate users are less likely to be flagged as suspicious. By integrating advanced fingerprinting with behavioral analysis, businesses can minimize disruptions caused by false positives. For example:
•
When a user switches between devices or networks, advanced fingerprinting ensures continuity in identification, avoiding unnecessary security prompts.
•
Tailored Fraud Detection for Emerging Threats
As fraud tactics grow more sophisticated—such as bot-driven attacks or coordinated fraud rings—advanced fingerprinting provides the adaptability needed to keep up. Techniques like link analysis map relationships between devices and accounts, uncovering patterns that indicate systemic fraud.
How CrossClassify’s Advanced Fingerprinting Technology Fights Fraud for Businesses
CrossClassify Fingerprint offers a cutting-edge device fingerprinting solution designed to generate unique hash codes for devices, enabling precise identification and detection of device sharing. By leveraging comprehensive device attributes and employing link analysis, CrossClassify creates similarity-based hash codes that enhance the accuracy of fraud detection and prevention. These codes play a crucial role in identifying suspicious account-device relationships and detecting shared or compromised devices
With its proprietary fingerprinting technology, CrossClassify integrates seamlessly into AI/ML fraud detection models. This empowers businesses to analyze critical features, such as the number of accounts linked to a single device or the number of devices used by one account. By ensuring precise and adaptable device identification, CrossClassify strengthens fraud detection strategies while minimizing disruptions for legitimate users.
Conclusion
Device fingerprinting has proven itself as a game-changing technology in the fight against fraud. Its ability to adapt to dynamic environments, analyze behavioral patterns, and integrate AI-driven techniques ensures precise device identification and enhanced security. By leveraging attributes ranging from hardware specifications to user interaction patterns, device fingerprinting minimizes false positives, detects sophisticated fraud schemes, and protects legitimate users without compromising their experience.
As businesses navigate the complexities of fraud prevention, technologies like CrossClassify’s advanced device fingerprinting provide the tools needed to stay one step ahead. To delve deeper into the workings of device fingerprinting and the data it collects, explore our detailed guide for further insights into this powerful solution.
Key Notes:
Introduction
How Device Fingerprinting Works?
What Information Is Collected to Create a Device Fingerprint?
Real-World Testing Scenarios for Fingerprinting
Privacy-Preserving Fingerprinting Approaches
How Advanced Device Fingerprints Revolutionize Fraud Detection
How CrossClassify’s Advanced Fingerprinting Technology Fights Fraud for Businesses
Conclusion
Share in
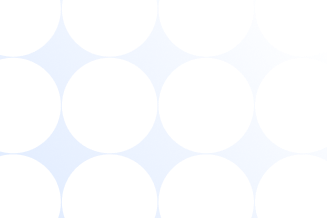
Let’s Get Started
Discover how to secure your app against fraud using CrossClassify
No credit card required